Copy link
Statistical tests: Sensitivity
Last updated: 03/04/2015
Sensitivity refers to the ability of a diagnostic modality (lab test, X-Ray etc.) to correctly identify all patients with the disease. It is defined as the ratio of the proportion of the patients who have the condition of interest and whose test results are positive over the number who have the disease. It is usually expressed as a percentage.
Consideration of this can be given by creating a 4×4 grid of test outcomes and disease states. Each square can be considered true or false (when comparing the test result to the disease status). (See Table 1).
Sensitivity can be calculated as shown in Table 2.
Sensitivity = Number of Patients with the Disease who Test Positive (True Positives)/Number of patients who have the disease (True Positives + False Negatives
Sensitivity = 90 / (90 + 10) = 0.9 or 90%
This has important implications, as a highly sensitive test (i.e. one whose sensitivity approaches 100%) is unlikely to be negative if a disease is present. In other words, a highly sensitive test with a negative result excludes the possibility of that disease.
As can be demonstrated above, reducing the number of false negatives can increase the sensitivity of a test. But it is important to note that a positive result from a highly sensitive test however, does not secure a diagnosis. It merely fails to exclude it. A test that always is positive (for all patients, at all times) has a sensitivity of 100% but is clearly clinically useless.
As the exact correlation between positive test results and presence of disease depends on both the performance characteristics of the test and the incidence of the disease in question from the population studied. This is a related concept called positive predictive value.
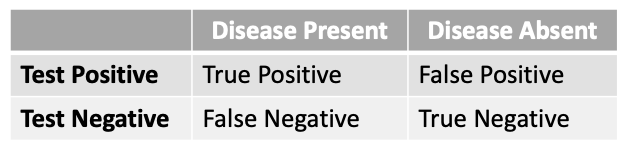
Table 1
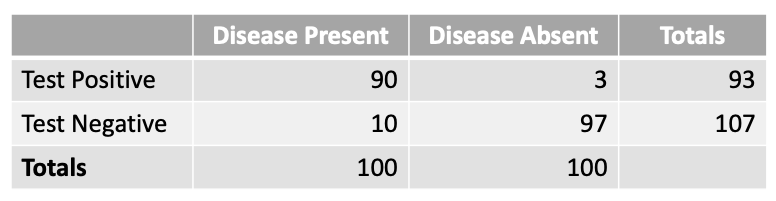
Table 2
Copyright Information
This work is licensed under a Creative Commons Attribution-NonCommercial-NoDerivatives 4.0 International License.